By Hans Dau - October 10, 2021
Executives are being bombarded with headlines about accelerating inflation, a phenomenon that many of the current generations of business leaders have never experienced firsthand.
Rather than trying to predict macroeconomic trends, executives of B2B companies are better served taking advantage of this environment of flux to implement long-overdue tactical pricing optimization initiatives with reduced risk of customer pushback.
Inflation or not?
PPI inflation has undeniably been significant at over 7% annually, even if measured from July 2029 to July 2021. While most agree that we're not in a 70s style price-wage inflation spiral yet, there is some valid concern. One camp of economists views current inflation as largely transitory, essentially an expected and desirable result of restarting the complex global supply chains that were disrupted by COVID-19. When COVID hit, producer prices declined by about 10% in Q1 2020 and have been on a steady uptrend since.
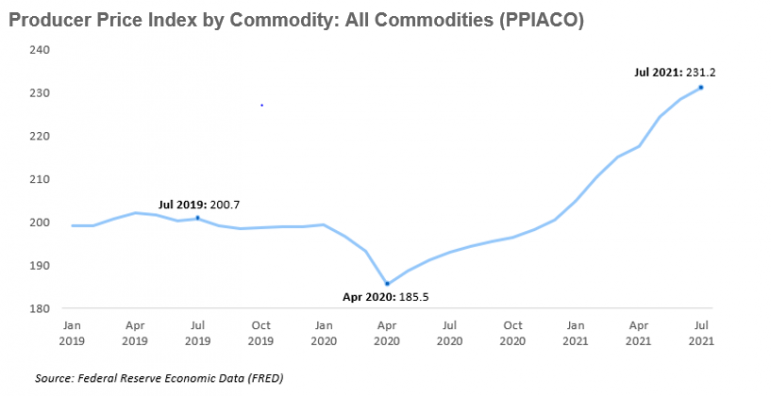
The other group of pundits is concerned about a combination of expansionary monetary policies and the potentially unprecedented fiscal stimulus in the form of various infrastructure bills and yet unspent COVID relief funds. The Fed's balance sheet doubled from an already high pre-pandemic $4 trillion to $8 trillion in only 12 months. While the Fed is projecting tighter policies by the end of 2023 with two projected interest rate increases, a lot can happen in the meantime.
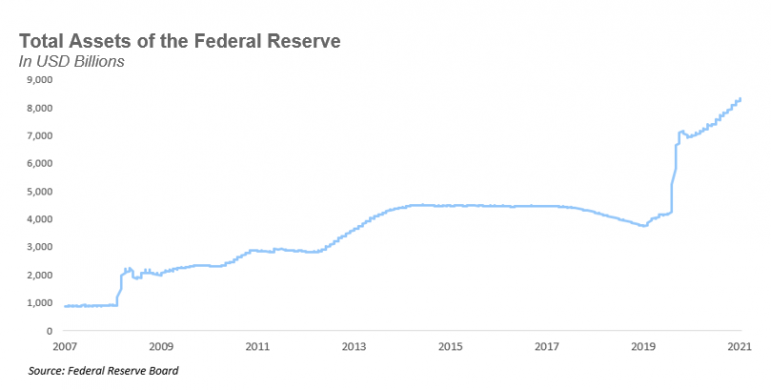
While both points of view have merit, corporate financial executives need to be prepared for either outcome and rethink their pricing strategy and tactics immediately. Clearly, customers will be far more receptive to price changes in an overall environment of adjustments across the global supply chain. This state of flux is the impetus for smart executives to act while the window of opportunity exists.
Pricing optimization imperative
Pricing is one of the most important drivers of bottom-line profitability and investing in better tactical pricing capabilities is a very high ROI proposition. Too often, pricing is viewed as static, macro market-driven and ignores easily obtainable data that contains highly relevant information content for tactical pricing decisions. Even if executives do not change overall price levels, the current environment has created an opportunity to implement tactical pricing optimization initiatives with less likelihood of adverse customer reactions than ever before.
I will focus on B2B transactional, i.e. tactical pricing here, which is where we see the most leverage in the current environment. At the most fundamental level, pricing is determined by the cost of production, degree of competition in the marketplace, and the shape of the demand curve, i.e. price elasticity. Most corporations have a good handle on cost, at least at the aggregate level, have a good understanding of competitive dynamics in key markets, but tend to ignore significant differences in customer sensitivity to the price that is often highly situational to a specific transaction.
The objective of analytical B2B pricing is to maximize the expected customer lifetime value by utilizing all available data, and applying information maximizing models to optimize the terms of every commercial transaction.
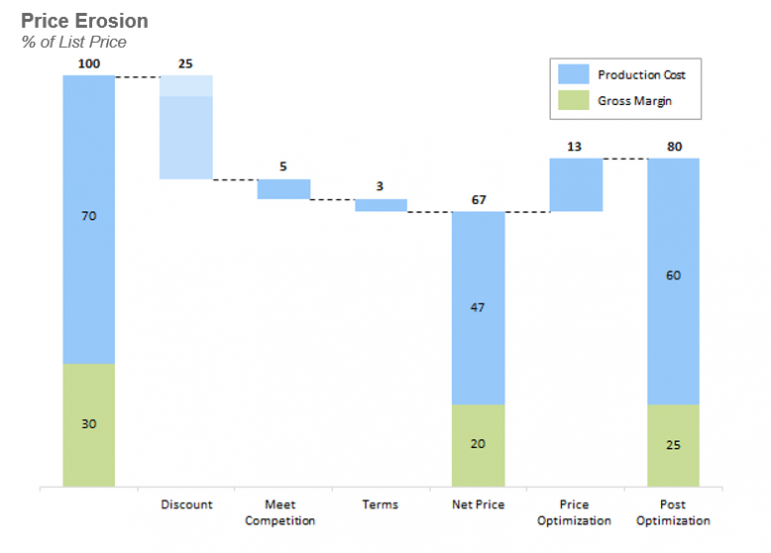
The impact of reversing the margin loss of price erosion can be enormous. In complex pricing environments (i.e. many products, geographies, distribution channels, large sales force, etc.) we have seen as much as 25% gross margin lift from pricing optimization.
Simple opportunity assessment check
There are simple and quick ways to analyze available CRM data to gauge the size of the opportunity and possibly pinpoint quick hit opportunities. For example, financial executives should ask the following basic questions:
Is there a consistent correlation between discounts and deal size?
Do sales executives quickly go to their maximum discount authority?
Is there a wide variation in discounts for deals of similar size?
Are discount levels arbitrary, i.e. in 5%, 10%, 15% bands?
Often, the answer to all these questions is yes, as illustrated by the below chart, indicating a significant opportunity for pricing optimization.
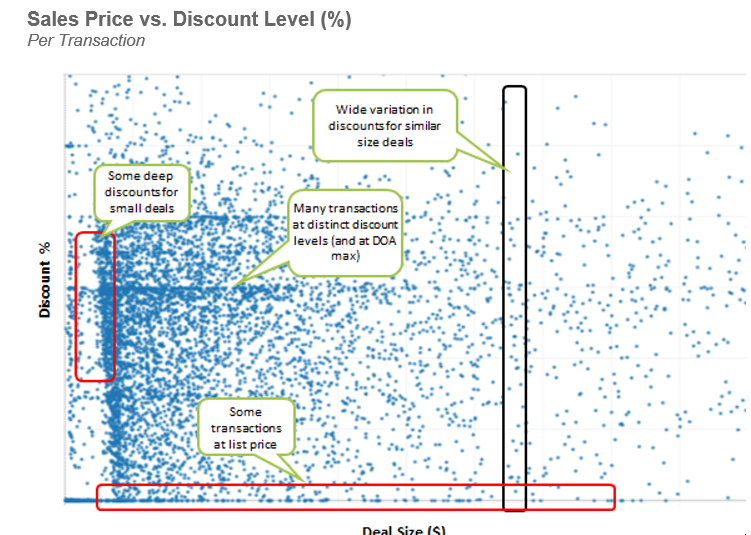
Running basic diagnostics on CRM data will likely also reveal other obvious issues that can be fixed quickly. There may be list pricing that is totally out of line (e.g. below cost), consistent discount abusing sales executives, loss-making customers, etc. all of which do not require sophisticated analysis to remedy.
The remainder of this article describes the typical steps required to create a sophisticated tactical pricing capability for sustained competitive advantage.
1. Assemble data with high information content.
It is critical to create a data set for subsequent machine learning that is consistent and likely to contain signals relevant to the pricing decision. The signal is likely to be multi-variant and non-obvious at this state, but at least the source of data should be logically related to the pricing challenge. Examples include social media data, blogs, emails that indicate social proximity between customers and employees of the corporation, sales pipeline data from the CRM system, channel promotions, purchases customer data, etc. It is less problematic to include low signal data as the models simply ignore it, but great care must be taken to make data consistent and understand any systematic biases that may have affected how the data was generated. For example, a CRM system may contain data from several pre-merger entities that had very different salesforce compensation policies. It is vitally important to understand such issues, and correct them.
Example variables used in machine learning models

Customer lifetime values maximization is at the heart of optimization. The goal should be to maximize expected customer lifetime value, i.e. one should be indifferent between a big/low probability and a small/high probability customer. Depending on the industry, customer LTV models may be more or less developed, but generally, they exist or can be created without too much difficulty. Creating clean outcome variables is often more challenging. Clean outcome coding is rare in CRM systems but critical for machine learning. A simple "Lost Sale" code in the CRM system could cover anything from "I will never buy anything from your company" to "We love your product but are out of budget for this quarter" and clearly produce a very poor classification model. CRM data needs to be cleaned and made consistent across different organizational units, products, markets, channels, and sales reps. In cases of very poor data but high transactions, it is often better to establish proper outcome and treatment coding and run the model with only three months of history rather than years of garbage data.
2. Create a robust customer LTV based outcome model

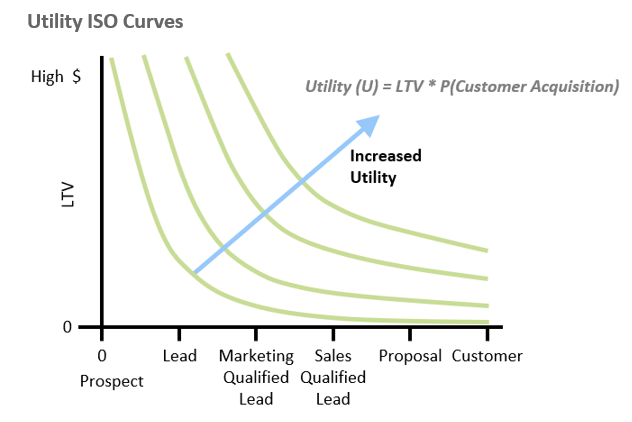
3. Perform analytics
Once the data is assembled and cleaned and clear objectives are established, the analytics will extract the information content and identify high signal variables. Proven analytical machine learning approaches focus on predicting which pipeline opportunities will result in sales success under various pricing scenarios. The end result is an opportunity score and a pricing recommendation for each specific customer transaction.
If the analytical capabilities do not exist in the organization, it should be hired from the outside. The machine learning part is a necessary but insufficient factor to success. The old "garbage in garbage out" mantra applies here. Unfortunately, we have seen many organizations place too much faith in sophisticated off-the-shelf software models that sit on top of poor data, leading to very poor results.

4. Interpret machine learning results
This is a critical step where art meets science to some extent. Firstly, interpreting the data may lead to uncovering unexpected data integrity issues that can be fixed at this stage. Machine learning by definition is hampered by a "black box" mentality, but an intuitive interpretation of the results is invaluable for implementation buy-in from the organization. Ideally, the interpretation of the machine learning output matches human intuition and confirms anecdotal evidence from the sales force. Taking the time to do this step helps greatly with implementing any recommendation throughout the organization compared to a black-box.

5. Implement recommendations.
This final typically required a combination of system and organizational changes to ensure effective implementation. The existing CRM system can usually be used to implement the model recommendations by simply feeding pricing recommendations from the model and scoring sales prospects. The exercise often unveils the differential performance of sales executives who are also likely to be incapable of implementing the new processes. In our experience, situations where half the sales force could reliably be predicted to close less than 10% of total sales, are common.

Conclusion
We believe that an analytical approach to pricing is one of the most important earnings drivers available to financial executives today. Cost-cutting may be difficult in a resource-constrained and inflationary environment, while simply raising prices across the board is risky and leaves money on the table compared to the analytical approach described here. Creative use of data and advanced analytical techniques enable smart executives to pinpoint the most desirable customer, price optimally and focus their sales resources effectively.
Comments